Your Success, Our Triumph
Data Science Management Achievements
Transport Industry
- Predictive Maintenance
- Routes Optimisation and Planning
- Fuel Consumption Optimisation and Control
Lineas, the larget train freight company in Europe, has a mandatory mid-life maintenance cycle for all its locomotives. They have a total of 200 locomotives. They do not all serve the same routes or do the same functions; some are used for shunting, while others travel short routes or long ones.
Based on the data collected from over 200 sensors on each locomotive, generating billions of records, we managed to compile a model that suggest the time after which each locomotive needed to undergo its “mid-life” maintenance cycle. Learn more about Lineas.
When averaging the results, we managed to extend the life-cycle per
locomotive by 15%. As a result, the savings generated are considerable.
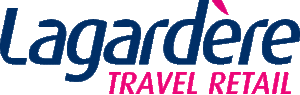
Retail
- Predictive Stock Management
- Shelves Assortment Optimisation
- Enhance Customer Experience
- Real Time Analytics meant to empower Store Managers
Lagardere Travel, part of Lagardere Group, owner of over 270 duty free shops across Europe, approached us to maximise their same store sales numbers.
After getting connected to their POS system and ERP, which allowed us to collect live data, we were able to uncover opportunities for them:
- Optimise assortment across the terminals
- Increase penetration in key customer segments
- Improve stores’ conversion rate
- Improve customer service (staffing optimisation)
- Empowered Store Managers with predictive and real-time analytics all the way to the items (SKU) level.
in their sales numbers in a period 90 days only/
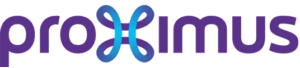
Telecommunications
& Infrastructure
- Precise Infrastructure Problem Identification
- Infrastructure Preventive Maintenance
- Identifying Problems before they occur
Proximus, the largest telecommunication operator in Belgium, had challenges to identify the problems on their network. In most of the cases, to address any given problem, they ought to send a technician onsite who do the standard troubleshooting procedure. If this technician could not identify/solve the problem, then they needed to dispatch a team who would have to dig a whole in the road and conduct a physical troubleshooting procedure.
Our Data Science Management have developed a model, that assembled thousand of data points per second and processed them. This has allowed us to predict correctly which technician is required to solve the trouble, we prevent tens of thousands of useless interventions per year. From field feedback, the algorithm is even a better predictor than technicians’ diagnostics.
Contact Form
Contact Us (FR)
"*" indicates required fields